Insights
Gain new perspectives on the key issues impacting eDiscovery, information governance, modern data, AI, and more. From the latest in innovative technology and AI to helpful tips and best practices, we’re here to shed a little light on what better looks like.
View all insightsFeatured Insights
Thank you! Your submission has been received!
Oops! Something went wrong while submitting the form.
eBook
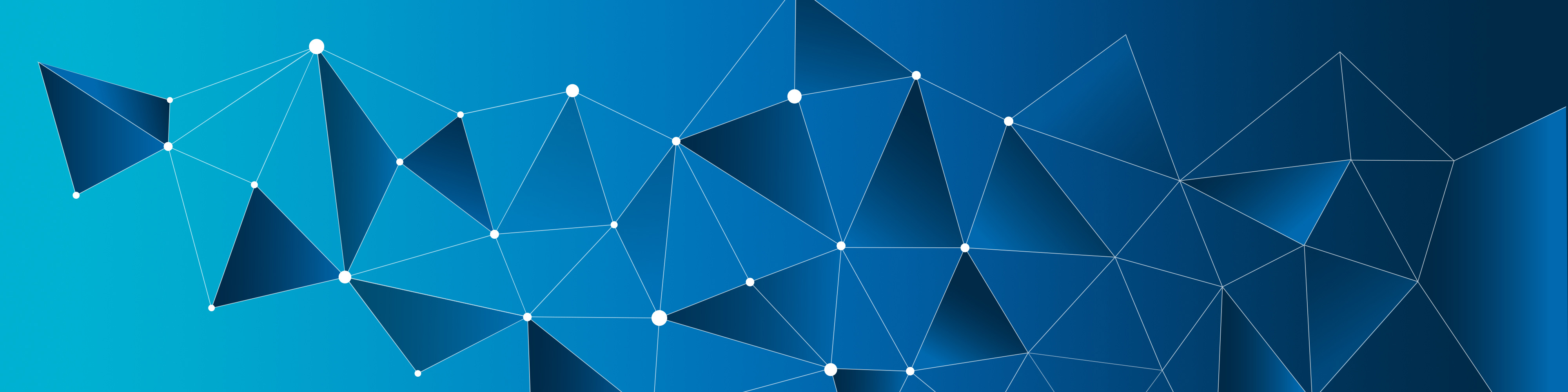
AI Is All the Rage — But What’s the ROI in eDiscovery?
[h2] Not All AI is Created Equally The eDiscovery market is suddenly crowded with AI tools and platforms. It makes sense—AI is perfectly suited for the large datasets, rule-based analysis, and need for speed and efficiency that define modern document review. But not all AI tools are created equally—so how do you sort through the noise to find the solutions best fit for you? What’s most important? The latest, greatest tech or what’s tried and true? At the end of the day, those aren’t the most important questions to consider. Instead, here are three questions you need to answer right away: What is my goal? How Is AI uniquely suited to help me? What are the measures of success? These questions will help you look beyond the “made with AI” labels and find solutions that make a real difference on your work and bottom line. To get you started, here are 4 ways that our clients have seen AI add value in eDiscovery. [h2] AI in eDiscovery: 4 ways to measure ROI Document review accuracy Risk mitigation Speed to strategy and completion Cost of eDiscovery [h2] AI Improves Document Review Deliverables and Timelines Studies have shown that machine learning tools from a decade ago are at least as reliable as human reviewers—and today’s AI tools are even better. Lighthouse has proven this in real-world, head-to-head comparisons between our modern AI and other review tools (see examples below). Analytic tools built with AI, such as large language models (LLMs), do a better job of detecting privilege, personally identifiable information, confidential information, and junk data. This saves a wealth of time and trouble down the line, through fewer downstream tasks like privilege review, redactions, and foreign language translation. It also significantly lowers the odds of disclosing non-relevant but sensitive information that could fuel more litigation. [h3] Document review accuracy [tab 1: open] Comparison [tab 2: closed] Examples No/Old AI Modern AI Words evaluated individually, at face value Words evaluated in context, accounting for different usages/meanings Analysis limited to text Analysis includes text, metadata, and other data types Broad analysis pulls in irrelevant docs for review Variable efficacy, highly dependent on document richness and training docs Nuanced analysis pulls in fewer irrelevant docs for review Specific base models for each classification type leads to more accurate analytic results [tab 1: closed] Comparison [tab 2: open] Examples Lighthouse AI Results in Smaller, More Precise Responsive Sets* During review for a Hart-Scott-Rodino Second Request, counsel ran the same documents through 3 different TAR models (Lighthouse AI, Relativity, and Brainspace) with the same training documents and parameters. *Data shown is for 70% recall. 308K fewer documents than Relativity; ~94K fewer than Brainspace 89% precision, compared to 73% for Relativity and 83% for Brainspace Lighthouse AI Outperforms Priv Terms In a matter with 1.5 million documents, a client compared the efficacy of Lighthouse AI and privilege terms. The percentage of potential privilege identified by each method was measured against families withheld or redacted for privilege. 8% privilege search terms 53% Lighthouse AI [h2] AI Mitigates Risk Through Data Reuse and Trend Analysis The accuracy of AI is one way it lowers risk. Another way is by applying knowledge across matters: Once a document is classified for one matter, reviewers can see how it was coded previously and make the same classification in current and future matters. This makes it much less likely that you’ll produce sensitive and privileged information to investigators and opposing counsel. Additionally, AI analytics are accessible in a dashboard view of an organization’s entire legal portfolio, helping teams identify risk trends they wouldn’t see otherwise. For example, analytics might show a higher incidence of litigation across certain custodians or a trend of outdated material stored in certain data sources. [h3] Risk mitigation [tab 1: open] Comparison [tab 2: closed] Examples No/Old AI Modern AI Search terms miss too many priv and sensitive docs Search terms cannot show historical coding Nuanced search finds more priv and sensitive docs Historical coding insights help reviewers with consistency Docs may be coded differently across matters, increasing risk of producing sensitive or priv docs Coding can be reused, increasing consistency and lowering risk QC relies on the same type of analysis as initial review (i.e., more humans) QC bolstered by statistical analysis; discrepancies between AI and attorney judgments indicate a need for more scrutiny [tab 1: closed] Comparison [tab 2: open] Examples Lighthouse AI Powers Consistency in Privilege Review A global pharmaceutical company asked Lighthouse to use advanced AI analytics on a group of related matters. This enabled the company to reuse a total of 26K previous privilege coding decisions, avoiding inadvertent disclosures and heading off potential challenges from opposing counsel. Reused priv coding Case A 4,300 Case B 6,080 Case C 970 Case D 4,100 Case E 11,000 [h2] AI Empowers with Early Insights and Faster Workflows Enhancements in AI technology in recent years have led to tools that work faster even when dealing with large datasets. They provide a clearer view of matters at an earlier stage in the game, so you can make more informed legal and strategy decisions right from the outset. They also get you to the end of document review more quickly, so you can avoid last-minute sprints and spend more time building your case. [h3] Speed to strategy and completion [tab 1: open] Comparison [tab 2: closed] Examples No/Old AI Modern AI Earliest insights emerge weeks to months into doc review Initial insights available within days for faster case assessment and data-backed case strategy Responsive review and priv review must happen in sequence Responsive review and priv review can happen simultaneously Responsive model goes back to start if the dataset changes Responsive models adapt to dataset changes False negatives lead to surprises in later stages No surprises QC spends more time managing review and checking work QC has more time to assess the substance of docs Review drags on for months Review completed in less time [tab 1: closed] Comparison [tab 2: open] Examples Lighthouse AI Crushes CAL for Early Insights Case planning and strategy hinge on how soon you can assess responsiveness and privilege. Standard workflows for advanced AI from Lighthouse are orders of magnitude faster than traditional CAL models. Dataset: 2M docs Building the responsive set Detecting sensitive info CAL & Regex 8 weeks 8+ weeks Lighthouse AI 15 days including 2 wks to train and 24 hrs to produce probability assessments (highly likely, highly unlikely, etc.) 24 hrs for arrival of first probability assessments [h2] AI Lowers eDiscovery Spend The accuracy, risk mitigation, and speed of advanced AI tools and analytics add up to less eyes-on review, faster timelines, and lower overall costs. [h3] Cost of eDiscovery [tab 1: open] Comparison [tab 2: closed] Examples No/Old AI Modern AI Excessive eyes-on review requires more attorneys and higher costs Eyes-on review can be strategically limited and assigned based on data that requires human decision making Doc review starts fresh with each matter Doc review informed and reduced by past decisions and insights Lower accuracy of analytics means more downstream review and associated costs Higher accuracy decreases downstream review and associated costs ROI limited by document thresholds and capacity for structured data only ROI enhanced by capacity for an astronomical number of datapoints across structured and unstructured data [tab 1: closed] Comparison [tab 2: open] Examples Lighthouse AI Trims $1M Off Privilege Review Costs In a recent matter, Lighthouse’s AI analytics rated 208K documents from the responsive set “highly unlikely” to be privileged. Rather than verify via eyes-on review, counsel opted to forward these docs directly to QC and production. In QC, reviewers agreed with Lighthouse AI’s assessment 99.1% of the time. 208K docs removed from priv review = $1.24M savings* *Based on human review at a rate of 25 docs/hr and $150/hr per reviewer. Lighthouse AI Significantly Reduces Eyes-On Review The superior accuracy of Lighthouse AI helped outside counsel reduce eyes-on review by identifying a smaller responsive set, removing thousands of irrelevant foreign-language documents, and targeting privilege docs more precisely. In terms of privilege, using AI instead of privilege terms avoided 18K additional hours of review. “My team saved the client $4 million in document review and translation costs vs. what we would have spent had we used Brainspace or Relativity Analytics.” —Head of eDiscovery innovation, Am Law 100 firm [h2] Finding the Right AI for the Job We hope this clarifies how AI can make a material difference in areas that matter most to you—as long as it’s the right AI. How can you tell whether an AI solution can help you accomplish your goals? Look for key attributes like: Large language models (LLMs) – LLMs are what enable the nuanced, context-conscious searches that make modern AI so accurate. Predictive AI – This is a type of LLM that makes predictions about responsiveness, privilege, and other classifications. Deep learning – This is the latest iteration of how AI gets smarter with use; it’s far more sophisticated than machine learning, which is an earlier iteration still used by many tools on the market. If you find AI terminology confusing, you’re not alone. Check out this infographic that provides simple, practical explanations. And for more information about AI designed with ROI in mind, visit our AI and analytics page below.
eBook
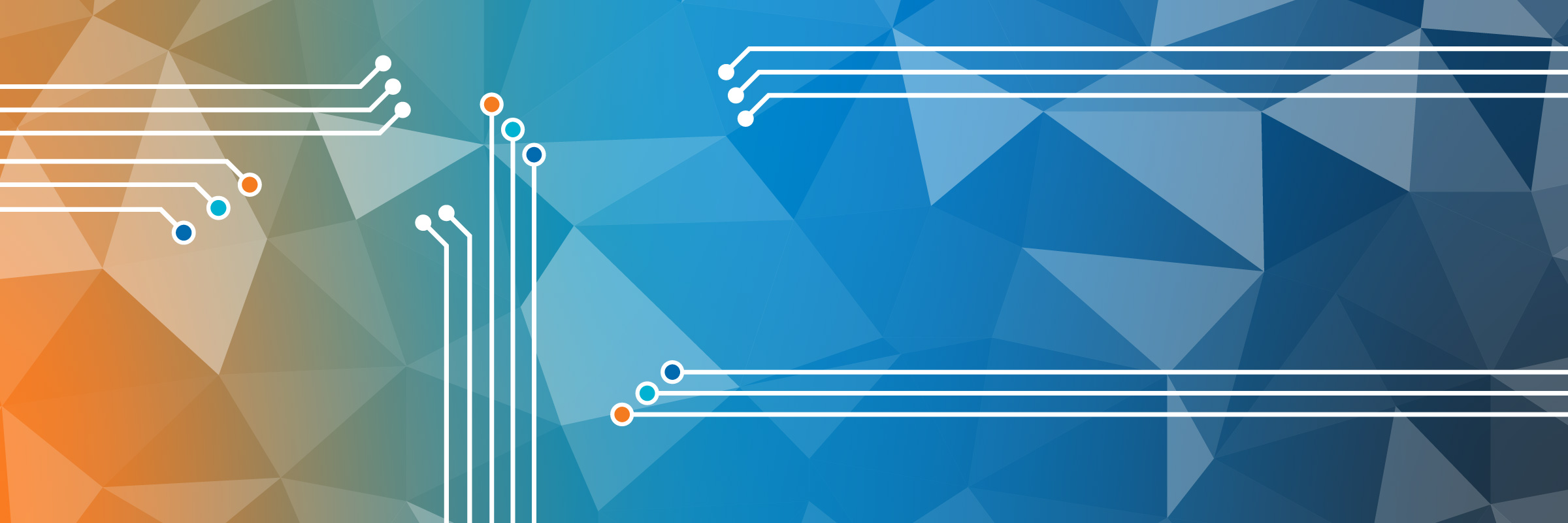
AI for eDiscovery: Terminology to Know
Everybody’s talking about AI. To help you follow the conversation, here’s a down-to-earth guide to the AI terms and concepts with the most immediate impact on document review and eDiscovery. Predictive AI. AI that predicts what is true now or in the future. Give predictive AI lots of data—about the weather, human illness, the shows people choose to stream—and it will make predictions about what else might be true or might happen next. These predictions are weighted by probability, which means predictive AI is concerned with the precision of its output. In eDiscovery: available now Tools with predictive AI use data from training sets and past matters to predict whether new documents fit the criteria for responsiveness, privilege, PII, and other classifications. Generative AI AI that generates new content based on examples of existing content ChatGPT is a famous example. It was trained on massive amounts of written content on the internet. When you ask it a question, you’re asking it to generate more written content. When it answers, it isn’t considering facts. It’s lining up words that it calculates will fulfill the request, without concern for precision. In eDiscovery: still emerging So far, we have seen chatbots enter the market. Eventually it may take many forms, such as creating a first draft of eDiscovery deliverables based on commands or prior inputs. Predictive AI and Generative AI are types of Large Language Models (LLMs) AI that analyzes language in the ways people actually use it LLMs treat words as interconnected pieces of data whose meaning changes depending on the context. For example, an LLM recognizes that “train” means something different in the phrases “I have a train to catch” and “I need to train for the marathon.” In eDiscovery: available but not universal Many document review tools and platforms use older forms of AI that aren’t built with LLMs. As a result, they miss the nuances of language and view every instance of a word like “train” equally. Ask an expert: Karl Sobylak, Director of Product Management, AI, Lighthouse What about “hallucinations”? This is a term for when generative AI produces written content that is false or nonsensical. The content may be grammatically correct, and the AI appears confident in what it’s saying. But the facts are all wrong. This can be humorous—but also quite damaging in legal scenarios. Luckily, we can control and safeguard against this. Where defensibility is concerned, we can ensure that AI models provide the same solution every time. At Lighthouse, we always pair technology with skilled experts, who deploy QC workflows to ensure precision and high-quality work product. What does this have to do with machine learning? Machine learning is the older form of AI used by traditional TAR models and many review tools that claim to use AI. These aren’t built with LLMs, so they miss the nuance of language and view words at face value. How does that compare to deep learning? Deep learning is the stage of AI that evolved out of machine learning. It’s much more sophisticated, drawing many more connections between data. Deep learning is what enables the multilayered analysis we see in LLMs.
No items found. Please try different search parameters.
Thank you! Your submission has been received!
Oops! Something went wrong while submitting the form.